Quantum computing, an emerging field that taps into the principles of quantum mechanics, is rapidly revealing its power to disrupt and revolutionize numerous sectors, not the least of which is optimization. Quantum optimization algorithms, like quantum annealing and quantum adiabatic optimization, are defining a new horizon in solving complex, high-stakes optimization problems that classical computers grapple with. The efficacy of these algorithms is deeply rooted in quantum phenomena such as why is quantum computing useful for optimization problems?, which confer quantum computers with an unrivaled computational advantage.
Unveiling the Quantum Advantage
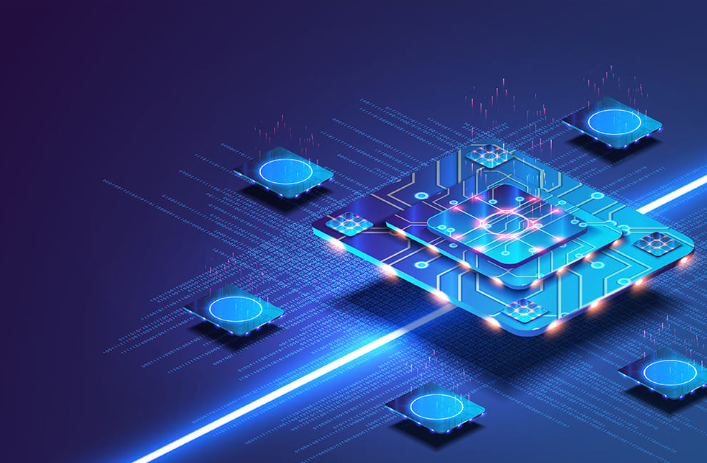
So, what sets quantum computing apart when it comes to optimization? The answer lies in its ability to leverage quantum superposition and quantum entanglement – strange, but fascinating properties exclusive to the quantum realm.
Superposition allows a quantum bit or qubit (the quantum counterpart of a classical bit) to exist in multiple states at once, unlike classical bits which can only be in a state of 0 or 1. This attribute allows quantum computers to perform multiple computations simultaneously, a trait known as quantum parallelism. The ability to process a multitude of potential solutions at once, rather than sequentially, makes quantum machines a perfect match for complex optimization tasks.
Quantum entanglement, another quantum phenomenon, further augments the computational prowess of quantum machines. In an entangled system, the state of one particle instantaneously affects the state of the other, regardless of the distance between them. This property can be exploited in optimization problems to achieve a global perspective, facilitating better and faster solutions.
Quantum Optimization Algorithms: A Quantum Leap for Optimization
The power of quantum computing is not just limited to its hardware capabilities but extends to quantum-specific algorithms as well. Two central players in the quantum optimization game are quantum annealing and quantum adiabatic optimization.
Quantum annealing uses quantum fluctuations to find the global minimum of an optimization problem – an aspect that classical annealing strategies often struggle with due to their propensity to get stuck in local minima. Quantum annealing thus can navigate the solution space more effectively, ensuring more accurate and optimal results.
Quantum adiabatic optimization, on the other hand, taps into the quantum adiabatic theorem. This principle posits that if a quantum system evolves slowly enough, it will stay close to its ground state (the state of lowest energy). Hence, starting from an easily solvable problem, the system can evolve into a final problem whose solution corresponds to the ground state, providing an optimal solution to the optimization problem.
The Quantum Revolution in Real-world Optimization Challenges
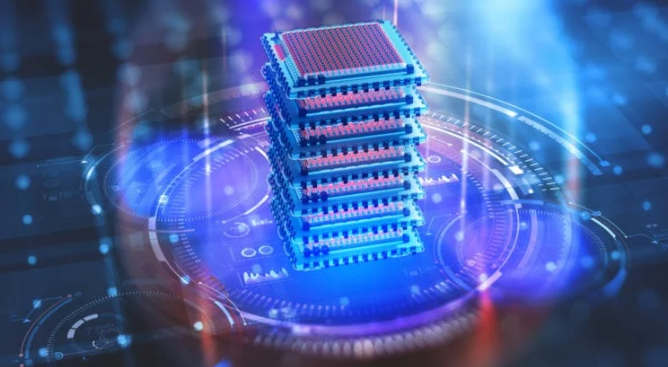
Quantum computing’s capacity for optimization is not limited to abstract problems but translates into tangible real-world applications. From optimizing logistics and supply chains to complex financial portfolios, and even refining machine learning models, the realm of quantum optimization is vast and impactful.
Take, for instance, the notorious Traveling Salesman Problem – a classical optimization problem that asks for the shortest possible route for a salesman to visit a set of cities and return to the original city. This problem falls into the category of NP-hard problems, which get exponentially harder to solve as the size of the problem increases. However, quantum computing holds a promising potential to solve such problems more efficiently, even as the problem size scales up, paving the way for quantum speedup.
The benefits of quantum computing also extend to constraint satisfaction problems, which are ubiquitous in AI, computer science, and operations research. The ability to find the most optimal solution while respecting a set of constraints makes quantum computing a game-changer in this area.
Harnessing Quantum Algorithms for Combinatorial Optimization
As we delve deeper into the quantum realm, another crucial application of quantum computing begins to surface – combinatorial optimization. This branch of optimization deals with finding the best object from a finite set of objects, with applications spanning fields such as artificial intelligence, logistics, telecommunication, and bioinformatics.
Complex problems such as the Knapsack problem or Job-shop scheduling, which are quintessential examples of combinatorial optimization problems, have traditionally been a challenge for classical computers. This is largely due to the vastness of the solution space, which grows exponentially with the size of the problem – a phenomenon termed combinatorial explosion.
Quantum computing, with its superior computational power, presents a novel way to tackle these issues. It leverages the principles of quantum superposition and quantum parallelism to process a multitude of potential solutions simultaneously, offering a significant speedup. Furthermore, quantum entanglement provides a way to maintain a correlation between different elements of a problem, thus facilitating a holistic approach to problem-solving, which is crucial in combinatorial optimization.
Quantum-inspired Optimization Techniques
While we eagerly await large-scale, fault-tolerant quantum computers, researchers have found ways to mimic quantum behavior on classical computers, leading to quantum-inspired optimization techniques. These techniques use concepts from quantum mechanics to design new algorithms for classical computers, giving us a sneak peek into the revolutionary potential of quantum optimization.
An example of this is the Quantum Approximate Optimization Algorithm (QAOA). It is a hybrid quantum-classical algorithm that applies a series of quantum and classical steps to gradually approximate the solution to combinatorial optimization problems. Despite being run on classical computers, the QAOA provides improved computational capabilities compared to traditional methods, signifying the potential of quantum-inspired techniques.
Quantum Computing and Large-scale Optimization Problems
A standout advantage of quantum computing is its potential to handle large-scale optimization problems. In many real-world applications, such as logistics and telecommunications, the optimization problems can involve hundreds or even thousands of variables. For classical computers, the computational cost of solving these problems scales unfavorably with problem size.
Quantum computers, on the other hand, can potentially offer an exponential speedup over classical algorithms. With quantum parallelism, a quantum computer can analyze all possible combinations of variables at once, dramatically reducing the time taken to find the optimal solution. Thus, for large-scale problems, the advent of quantum computing could mark a paradigm shift, drastically accelerating and improving optimization processes across a range of industries.
Quantum Computing: The Future of Optimization?
In conclusion, the immense computational power of quantum computing holds the potential to revolutionize the field of optimization. With abilities such as processing multiple solutions simultaneously, maintaining a global perspective on problem-solving, and managing large-scale problems effectively, quantum computing is setting new standards in optimization.
While challenges in quantum computing technology remain, the progress thus far is inspiring and foreshadows an exciting future. With advancements in quantum algorithms and quantum-inspired techniques, the application of quantum computing in optimization is not a distant dream but an impending reality.
Optimization forms the backbone of decision-making in numerous sectors – from logistics to artificial intelligence, telecommunications to financial services, and many more. As such, the prospect of quantum computing in enhancing optimization solutions bears enormous potential to reshape these sectors, opening up new frontiers in efficiency and problem-solving.
However, quantum computing is not just about better solutions, but about solutions that were previously thought to be unattainable. As we continue to explore this exciting field, we stand on the brink of a new era of optimization, powered by quantum computing.
Also Read: How is Accenture Addressing the Emerging Market for Quantum Computing Technology?
Final Thoughts: Why is Quantum Computing Useful for Optimization Problems?
Quantum computing indeed holds immense promise in solving complex optimization problems. However, it’s important to note that we are still in the early stages of quantum computing technology. Challenges in maintaining quantum coherence and dealing with quantum noise persist, and quantum computers with a sufficient number of qubits for widespread practical use are yet to be developed.
Yet, the progress made so far is encouraging. As the field matures, we can expect an exponential increase in computational capabilities that will redefine the way we approach optimization problems. This new quantum era holds the promise of not just incrementally better solutions, but a paradigm shift in our problem-solving capabilities.
In a nutshell, the combination of quantum parallelism, quantum superposition, quantum annealing, and quantum adiabatic optimization is reshaping the landscape of complex problem-solving, placing quantum computing at the heart of the next big leap in optimization solutions. As we dive deeper into the quantum realm, the possibilities seem not just promising, but limitless.